R1-AQA
Xiaomi's DeepSeek-R1 Inspired Audio AI
Listed in categories:
Open SourceAudioArtificial Intelligence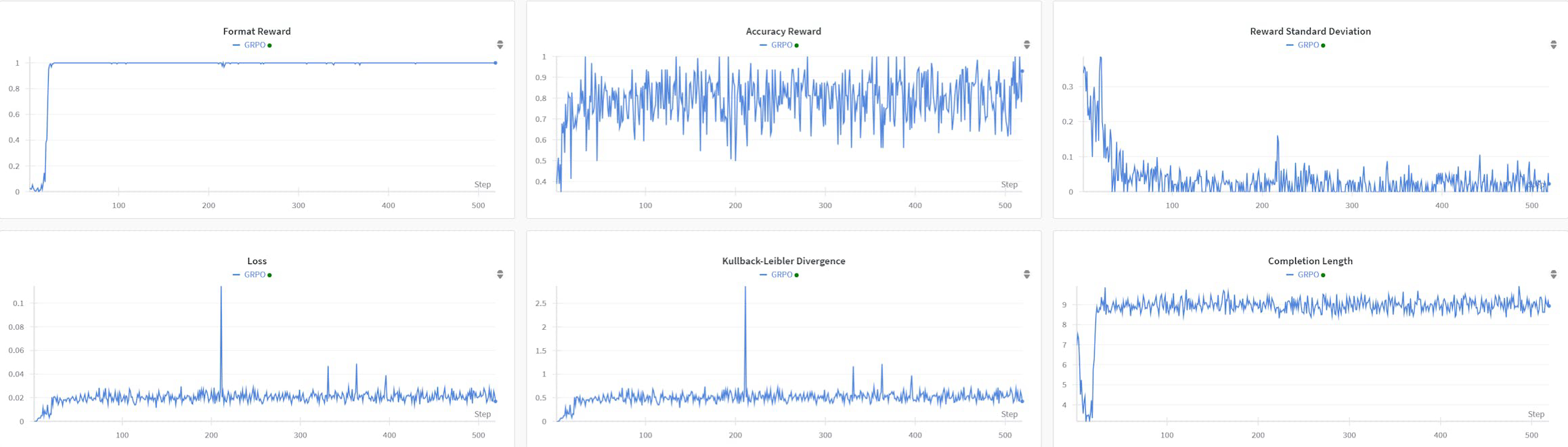
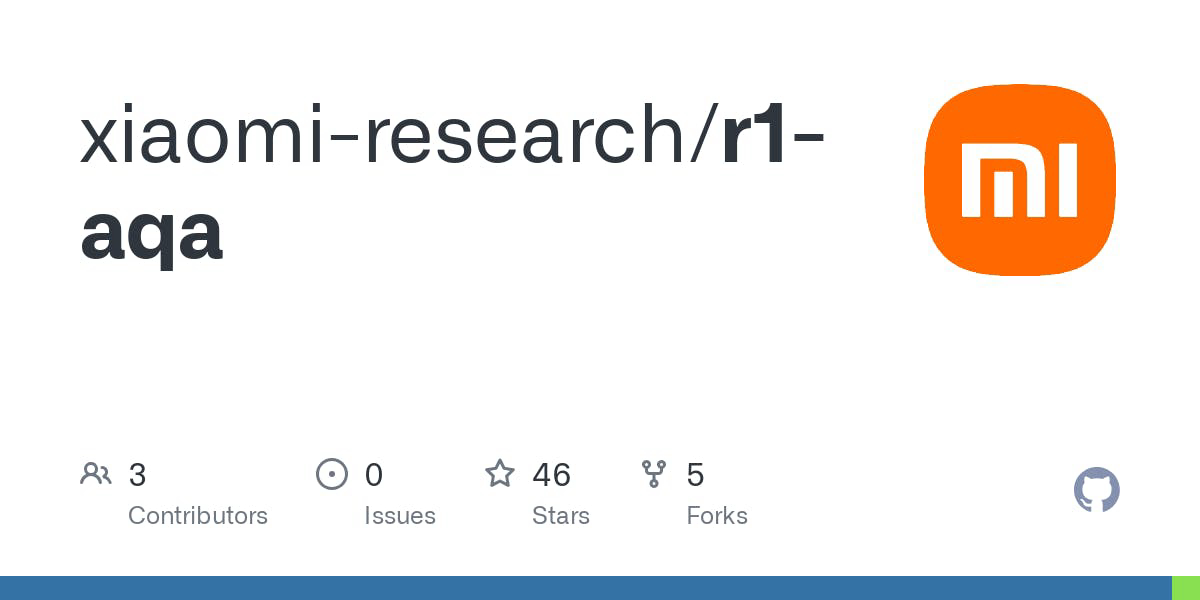
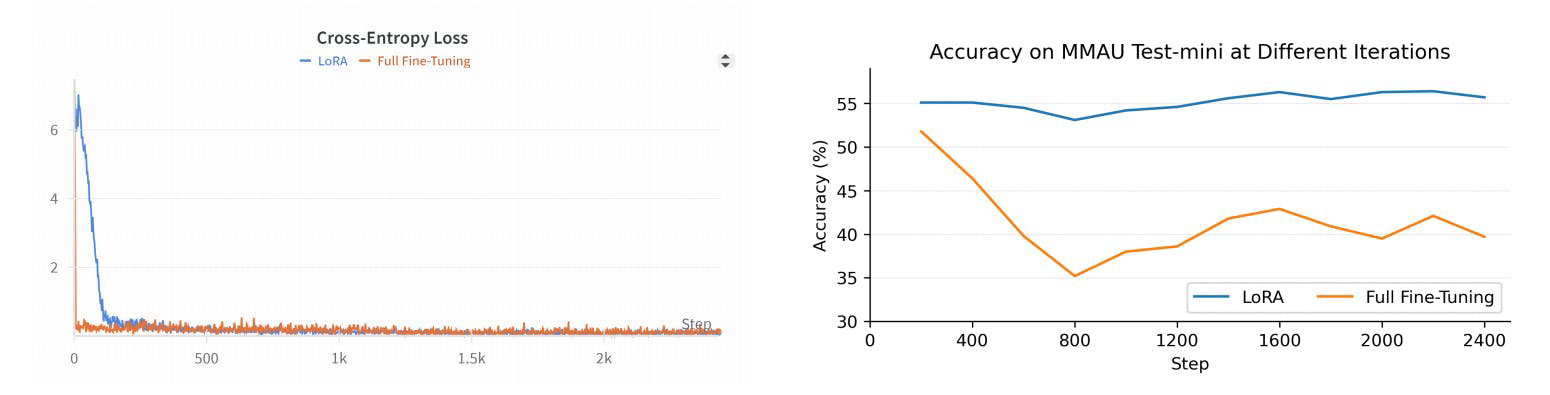
Description
R1AQA is an advanced audio question answering (AQA) model based on Qwen2Audio7BInstruct, optimized through reinforcement learning (RL) using the group relative policy optimization (GRPO) algorithm. It has achieved state-of-the-art performance on the MMAU Testmini benchmark with only 38k post-training samples, demonstrating the effectiveness of RL-based approaches in AQA tasks without the need for large datasets.
How to use R1-AQA?
To use R1AQA, download the model and follow the provided instructions to prepare your dataset. You can then run the evaluation scripts to test the model's performance on audio question answering tasks.
Core features of R1-AQA:
1️⃣
State-of-the-art performance on audio question answering tasks
2️⃣
Optimized using reinforcement learning techniques
3️⃣
Utilizes the group relative policy optimization algorithm
4️⃣
Requires only a small number of post-training samples
5️⃣
Supports various audio modalities for question answering
Why could be used R1-AQA?
# | Use case | Status | |
---|---|---|---|
# 1 | Enhancing audio-based search engines | ✅ | |
# 2 | Improving accessibility features for audio content | ✅ | |
# 3 | Developing interactive audio learning tools | ✅ |
Who developed R1-AQA?
The R1AQA model is developed by a team of researchers including Gang Li, Jizhong Liu, Heinrich Dinkel, Yadong Niu, Junbo Zhang, and Jian Luan, who have made significant contributions to the field of audio question answering and reinforcement learning.